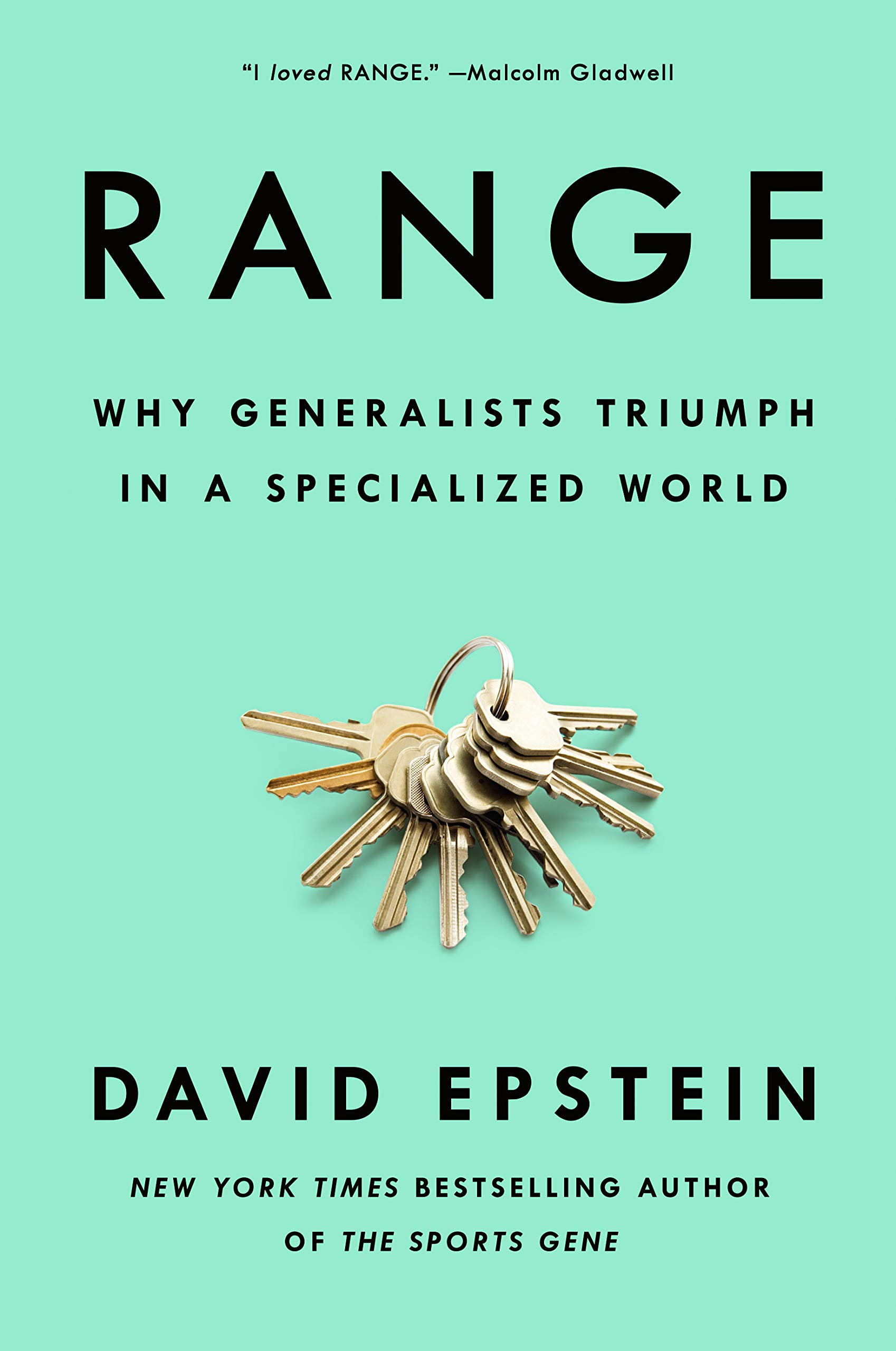
Range: Why Generalists Triumph in a Specialized World
von Dabid Epstein
And he refused to specialize in anything, preferring to keep an eye on the overall estate rather than any of its parts. . . . And Nikolay’s management produced the most brilliant results. —Leo Tolstoy, War and Peace
Eventual elites typically devote less time early on to deliberate practice in the activity in which they will eventually become experts. Instead, they undergo what researchers call a “sampling period.” They play a variety of sports, usually in an unstructured or lightly structured environment; they gain a range of physical proficiencies from which they can draw; they learn about their own abilities and proclivities; and only later do they focus in and ramp up technical practice in one area.
I dove into work showing that highly credentialed experts can become so narrow-minded that they actually get worse with experience, even while becoming more confident—a dangerous combination. And I was stunned when cognitive psychologists I spoke with led me to an enormous and too often ignored body of work demonstrating that learning itself is best done slowly to accumulate lasting knowledge, even when that means performing poorly on tests of immediate progress. That is, the most effective learning looks inefficient; it looks like falling behind.
The challenge we all face is how to maintain the benefits of breadth, diverse experience, interdisciplinary thinking, and delayed concentration in a world that increasingly incentivizes, even demands, hyperspecialization.
While it is undoubtedly true that there are areas that require individuals with Tiger’s precocity and clarity of purpose, as complexity increases—as technology spins the world into vaster webs of interconnected systems in which each individual only sees a small part—we also need more Rogers: people who start broad and embrace diverse experiences and perspectives while they progress. People with range.
Whether or not experience inevitably led to expertise, they agreed, depended entirely on the domain in question. Narrow experience made for better chess and poker players and firefighters, but not for better predictors of financial or political trends, or of how employees or patients would perform.
In wicked domains, the rules of the game are often unclear or incomplete, there may or may not be repetitive patterns and they may not be obvious, and feedback is often delayed, inaccurate, or both.
Moravec’s paradox: machines and humans frequently have opposite strengths and weaknesses.
He studied high-powered consultants from top business schools for fifteen years, and saw that they did really well on business school problems that were well defined and quickly assessed. But they employed what Argyris called single-loop learning, the kind that favors the first familiar solution that comes to mind. Whenever those solutions went wrong, the consultant usually got defensive. Argyris found their “brittle personalities” particularly surprising given that “the essence of their job is to teach others how to do things differently.”
If the amount of early, specialized practice in a narrow area were the key to innovative performance, savants would dominate every domain they touched, and child prodigies would always go on to adult eminence. As psychologist Ellen Winner, one of the foremost authorities on gifted children, noted, no savant has ever been known to become a “Big-C creator,” who changed their field.
Kahneman pointed to those domains’ “robust statistical regularities.” But when the rules are altered just slightly, it makes experts appear to have traded flexibility for narrow skill. In research in the game of bridge where the order of play was altered, experts had a more difficult time adapting to new rules than did nonexperts. When experienced accountants were asked in a study to use a new tax law for deductions that replaced a previous one, they did worse than novices. Erik Dane, a Rice University professor who studies organizational behavior, calls this phenomenon “cognitive entrenchment.” His suggestions for avoiding it are about the polar opposite of the strict version of the ten-thousand-hours school of thought: vary challenges within a domain drastically, and, as a fellow researcher put it, insist on “having one foot outside your world.”
The main conclusion of work that took years of studying scientists and engineers, all of whom were regarded by peers as true technical experts, was that those who did not make a creative contribution to their field lacked aesthetic interests outside their narrow area.
Or electrical engineer Claude Shannon, who launched the Information Age thanks to a philosophy course he took to fulfill a requirement at the University of Michigan. In it, he was exposed to the work of self-taught nineteenth-century English logician George Boole, who assigned a value of 1 to true statements and 0 to false statements and showed that logic problems could be solved like math equations. It resulted in absolutely nothing of practical importance until seventy years after Boole passed away, when Shannon did a summer internship at AT&T’s Bell Labs research facility. There he recognized that he could combine telephone call-routing technology with Boole’s logic system to encode and transmit any type of information electronically. It was the fundamental insight on which computers rely. “It just happened that no one else was familiar with both those fields at the same time,” Shannon said.
They “traveled on an eight-lane highway,” he wrote, rather than down a single-lane one-way street. They had range. The successful adapters were excellent at taking knowledge from one pursuit and applying it creatively to another, and at avoiding cognitive entrenchment. They employed what Hogarth called a “circuit breaker.” They drew on outside experiences and analogies to interrupt their inclination toward a previous solution that may no longer work. Their skill was in avoiding the same old patterns. In the wicked world, with ill-defined challenges and few rigid rules, range can be a life hack.
The more they had moved toward modernity, the more powerful their abstract thinking, and the less they had to rely on their concrete experience of the world as a reference point.
In Flynn’s terms, we now see the world through “scientific spectacles.” He means that rather than relying on our own direct experiences, we make sense of reality through classification schemes, using layers of abstract concepts to understand how pieces of information relate to one another. We have grown up in a world of classification schemes totally foreign to the remote villagers; we classify some animals as mammals, and inside of that class make more detailed connections based on the similarity of their physiology and DNA.
Words that represent concepts that were previously the domain of scholars became widely understood in a few generations. The word “percent” was almost absent from books in 1900. By 2000 it appeared about once every five thousand words. (This chapter is 5,500 words long.) Computer programmers pile layers of abstraction. (They do very well on Raven’s.) In the progress bar on your computer screen that fills up to indicate a download, abstractions are legion, from the fundamental—the programming language that created it is a representation of binary code, the raw 1s and 0s the computer uses—to the psychological: the bar is a visual projection of time that provides peace of mind by estimating the progress of an immense number of underlying activities.
Modern work demands knowledge transfer: the ability to apply knowledge to new situations and different domains. Our most fundamental thought processes have changed to accommodate increasing complexity and the need to derive new patterns rather than rely only on familiar ones. Our conceptual classification schemes provide a scaffolding for connecting knowledge, making it accessible and flexible.
Each of twenty test questions gauged a form of conceptual thinking that can be put to widespread use in the modern world. For test items that required the kind of conceptual reasoning that can be gleaned with no formal training—detecting circular logic, for example—the students did well. But in terms of frameworks that can best put their conceptual reasoning skills to use, they were horrible. Biology and English majors did poorly on everything that was not directly related to their field. None of the majors, including psychology, understood social science methods. Science students learned the facts of their specific field without understanding how science should work in order to draw true conclusions. Neuroscience majors did not do particularly well on anything. Business majors performed very poorly across the board, including in economics. Econ majors did the best overall. Economics is a broad field by nature, and econ professors have been shown to apply the reasoning principles they’ve learned to problems outside their area.* Chemists, on the other hand, are extraordinarily bright, but in several studies struggled to apply scientific reasoning to nonchemistry problems.
When he recounts his own education at the University of Chicago, where he was captain of the cross-country team, he raises his voice. “Even the best universities aren’t developing critical intelligence,” he told me. “They aren’t giving students the tools to analyze the modern world, except in their area of specialization. Their education is too narrow.” He does not mean this in the simple sense that every computer science major needs an art history class, but rather that everyone needs habits of mind that allow them to dance across disciplines.
Jeannette Wing, a computer science professor at Columbia University and former corporate vice president of Microsoft Research, has pushed broad “computational thinking” as the mental Swiss Army knife. She advocated that it become as fundamental as reading, even for those who will have nothing to do with computer science or programming. “Computational thinking is using abstraction and decomposition when attacking a large complex task,” she wrote. “It is choosing an appropriate representation for a problem.”
Unsurprisingly, Fermi problems were a topic in the “Calling Bullshit” course. It used a deceptive cable news report as a case study to demonstrate “how Fermi estimation can cut through bullshit like a hot knife through butter.” It gives anyone consuming numbers, from news articles to advertisements, the ability quickly to sniff out deceptive stats. That’s a pretty handy hot butter knife. I would have been a much better researcher in any domain, including Arctic plant physiology, had I learned broadly applicable reasoning tools rather than the finer details of Arctic plant physiology.
They were perfectly capable of learning from experience, but failed at learning without experience. And that is what a rapidly changing, wicked world demands—conceptual reasoning skills that can connect new ideas and work across contexts.
That is not an option for us. The more constrained and repetitive a challenge, the more likely it will be automated, while great rewards will accrue to those who can take conceptual knowledge from one problem or domain and apply it in an entirely new one.
In totality, the picture is in line with a classic research finding that is not specific to music: breadth of training predicts breadth of transfer. That is, the more contexts in which something is learned, the more the learner creates abstract models, and the less they rely on any particular example. Learners become better at applying their knowledge to a situation they’ve never seen before, which is the essence of creativity.
“It’s strange,” Cecchini told me at the end of one of our hours-long discussions, “that some of the greatest musicians were self-taught or never learned to read music. I’m not saying one way is the best, but now I get a lot of students from schools that are teaching jazz, and they all sound the same. They don’t seem to find their own voice. I think when you’re self-taught you experiment more, trying to find the same sound in different places, you learn how to solve problems.”
Kornell was explaining the concept of “desirable difficulties,” obstacles that make learning more challenging, slower, and more frustrating in the short term, but better in the long term. Excessive hint-giving, like in the eighth-grade math classroom, does the opposite; it bolsters immediate performance, but undermines progress in the long run. Several desirable difficulties that can be used in the classroom are among the most rigorously supported methods of enhancing learning, and the engaging eighth-grade math teacher accidentally subverted all of them in the well-intended interest of before-your-eyes progress.
One of those desirable difficulties is known as the “generation effect.” Struggling to generate an answer on your own, even a wrong one, enhances subsequent learning. Socrates was apparently on to something when he forced pupils to generate answers rather than bestowing them. It requires the learner to intentionally sacrifice current performance for future benefit.
It isn’t bad to get an answer right while studying. Progress just should not happen too quickly, unless the learner wants to end up like Oberon (or, worse, Macduff), with a knowledge mirage that evaporates when it matters most. As with excessive hint-giving, it will, as a group of psychologists put it, “produce misleadingly high levels of immediate mastery that will not survive the passage of substantial periods of time.” For a given amount of material, learning is most efficient in the long run when it is really inefficient in the short run. If you are doing too well when you test yourself, the simple antidote is to wait longer before practicing the same material again, so that the test will be more difficult when you do. Frustration is not a sign you are not learning, but ease is.
In a study using college math problems, students who learned in blocks—all examples of a particular type of problem at once—performed a lot worse come test time than students who studied the exact same problems but all mixed up. The blocked-practice students learned procedures for each type of problem through repetition. The mixed-practice students learned how to differentiate types of problems.
The same effect has appeared among learners studying everything from butterfly species identification to psychological-disorder diagnosis. In research on naval air defense simulations, individuals who engaged in highly mixed practice performed worse than blocked practicers during training, when they had to respond to potential threat scenarios that became familiar over the course of the training. At test time, everyone faced completely new scenarios, and the mixed-practice group destroyed the blocked-practice group.
Kind learning environment experts choose a strategy and then evaluate; experts in less repetitive environments evaluate and then choose.
Mention Kepler if you want to get Northwestern University psychologist Dedre Gentner excited. She gesticulates. Her tortoiseshell glasses bob up and down. She is probably the world’s foremost authority on analogical thinking. Deep analogical thinking is the practice of recognizing conceptual similarities in multiple domains or scenarios that may seem to have little in common on the surface. It is a powerful tool for solving wicked problems, and Kepler was an analogy addict, so Gentner is naturally very fond of him. When she mentions a trivial historical detail about him that might be misunderstood by modern readers, she suggests that maybe it’s best not to publish it as it might make him look bad, though he has been dead for nearly four hundred years.
Those results are from a series of 1980s analogical thinking studies. Really, don’t feel bad if you didn’t get it. In a real experiment you would have taken more time, and whether you got it or not is unimportant. The important part is what it shows about problem solving. A gift of a single analogy from a different domain tripled the proportion of solvers who got the radiation problem. Two analogies from disparate domains gave an even bigger boost. The impact of the fortress story alone was as large as if solvers were just straight out told this guiding principle: “If you need a large force to accomplish some purpose, but are prevented from applying such a force directly, many smaller forces applied simultaneously from different directions may work just as well.”
Human intuition, it appears, is not very well engineered to make use of the best tools when faced with what the researchers called “ill-defined” problems. Our experience-based instincts are set up well for Tiger domains, the kind world Gentner described, where problems and solutions repeat.
In a wicked world, relying upon experience from a single domain is not only limiting, it can be disastrous.
For a unique 2012 experiment, University of Sydney business strategy professor Dan Lovallo—who had conducted inside-view research with Kahneman—and a pair of economists theorized that starting out by making loads of diverse analogies, Kepler style, would naturally lead to the outside view and improve decisions. They recruited investors from large private equity firms who consider a huge number of potential projects in a variety of domains. The researchers thought the investors’ work might naturally lend itself to the outside view.
This is a widespread phenomenon. If you’re asked to predict whether a particular horse will win a race or a particular politician will win an election, the more internal details you learn about any particular scenario—physical qualities of the specific horse, the background and strategy of the particular politician—the more likely you are to say that the scenario you are investigating will occur.
In one famous study, participants judged an individual as more likely to die from “heart disease, cancer, or other natural causes” than from “natural causes.” Focusing narrowly on many fine details specific to a problem at hand feels like the exact right thing to do, when it is often exactly wrong.
Seth Godin, author of some of the most popular career writing in the world, wrote a book disparaging the idea that “quitters never win.” Godin argued that “winners”—he generally meant individuals who reach the apex of their domain—quit fast and often when they detect that a plan is not the best fit, and do not feel bad about it. “We fail,” he wrote, when we stick with “tasks we don’t have the guts to quit.” Godin clearly did not advocate quitting simply because a pursuit is difficult. Persevering through difficulty is a competitive advantage for any traveler of a long road, but he suggested that knowing when to quit is such a big strategic advantage that every single person, before undertaking an endeavor, should enumerate conditions under which they should quit. The important trick, he said, is staying attuned to whether switching is simply a failure of perseverance, or astute recognition that better matches are available.
The computer world has a name for this: premature optimization. . . . . . . Instead of working back from a goal, work forward from promising situations. This is what most successful people actually do anyway.
Tetlock decided to put expert predictions to the test. With the Cold War in full swing, he began a study to collect short- and long-term forecasts from 284 highly educated experts (most had doctorates) who averaged more than twelve years of experience in their specialties. The questions covered international politics and economics, and in order to make sure the predictions were concrete, the experts had to give specific probabilities of future events. Tetlock had to collect enough predictions over enough time that he could separate lucky and unlucky streaks from true skill. The project lasted twenty years, and comprised 82,361 probability estimates about the future. The results limned a very wicked world. The average expert was a horrific forecaster. Their areas of specialty, years of experience, academic degrees, and even (for some) access to classified information made no difference. They were bad at short-term forecasting, bad at long-term forecasting, and bad at forecasting in every domain. When experts declared that some future event was impossible or nearly impossible, it nonetheless occurred 15 percent of the time.
Many experts never admitted systematic flaws in their judgment, even in the face of their results. When they succeeded, it was completely on their own merits—their expertise clearly enabled them to figure out the world. When they missed wildly, it was always a near miss; they had certainly understood the situation, they insisted, and if just one little thing had gone differently, they would have nailed it. Or, like Ehrlich, their understanding was correct; the timeline was just a bit off. Victories were total victories, and defeats were always just a touch of bad luck away from having been victories too. Experts remained undefeated while losing constantly. “There is often a curiously inverse relationship,” Tetlock concluded, “between how well forecasters thought they were doing and how well they did.”
The integrators outperformed their colleagues on pretty much everything, but they especially trounced them on long-term predictions. Eventually, Tetlock conferred nicknames (borrowed from philosopher Isaiah Berlin) that became famous throughout the psychology and intelligence-gathering communities: the narrow-view hedgehogs, who “know one big thing,” and the integrator foxes, who “know many little things.”
colleagues and clients, from refugees seeking asylum to Silicon Valley billionaires whom he would chat with
In separate work, from 2000 to 2010 German psychologist Gerd Gigerenzer compiled annual dollar-euro exchange rate predictions made by twenty-two of the most prestigious international banks—Barclays, Citigroup, JPMorgan Chase, Bank of America Merrill Lynch, and others. Each year, every bank predicted the end-of-year exchange rate. Gigerenzer’s simple conclusion about those projections, from some of the world’s most prominent specialists: “Forecasts of dollar-euro exchange rates are worthless.” In six of the ten years, the true exchange rate fell outside the entire range of all twenty-two bank forecasts. Where a superforecaster quickly highlighted a change in exchange rate direction that confused him, and adjusted, major bank forecasts missed every single change of direction in the decade Gigerenzer analyzed.